Cheramie, G. M., Schanding, G. T., & Streich, K. (2018). Core Selective Evaluation Process (C-SEP) and dual discrepancy/consistency models for LD identification: A case study approach. In D. Flanagan & V. Alfonso (Eds.), Essentials of specific learning disability identification (2nd ed., pp. 475–501). Hoboken, NJ: John Wiley & Sons.
Summary by Dr. Jack M. Fletcher
Overview and Key Findings
Methods for the identification of individuals with learning disabilities (LD) are hotly debated and controversial. This is especially true for methods based on patterns of strengths and weaknesses (PSW methods), an umbrella term touted as an alternative to cognitive discrepancy approaches based on aptitude-achievement discrepancies. PSW methods are often proposed in conjunction with methods based on response to intervention, and most proponents recommend assessment via PSW methods after inadequate response to Tier 2 intervention to determine whether lack of adequate response is due to LD.
In their chapter, Cheramie, Schanding, and Streich (2018) do not discuss when PSW assessments should take place (see Hale et al., 2010). However, they do present two PSW methods as approaches to LD identification. The “new” method is the Core Selective Evaluation Process (C-SEP) developed by Schultz and Stephens (2015), which is compared to the dual discrepancy/consistency method (DD/C; often referred to as the “cross-battery” method), an older approach developed by Flanagan and associates (Flanagan et al., 2018). The C-SEP has many parallels to DD/C but reduces the amount of testing and uses only subtests from the Woodcock-Johnson IV (WJ IV) tests of achievement, cognitive abilities, and oral language.
Figure 1. Illustration of the Identification Criteria of PSW Methods.
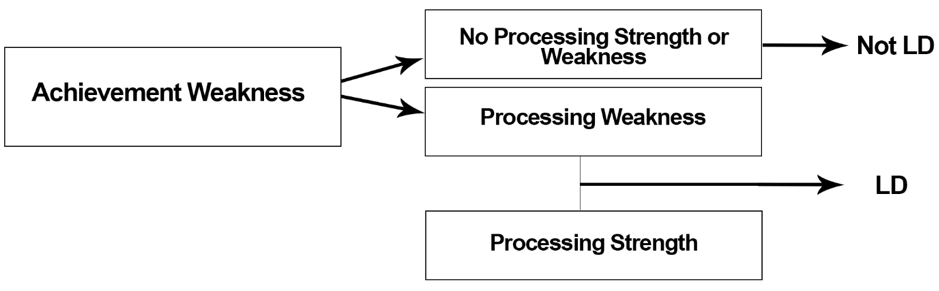
Cheramie et al. (2018) note that PSW methods share common features, including a weakness in achievement, a strength in cognitive processing, and a weakness in cognitive processing that is linked to the achievement weakness. This approach can be seen in Figure 1, which shows that when a person has the necessary achievement weakness, cognitive strength, and cognitive weakness, they can be identified with LD. Additional steps include meeting other traditional criteria that indicate that the achievement deficit is not primarily due to factors considered exclusionary of LD (e.g., intellectual disability, sensory disorder, emotional problems, cultural factors, economic disadvantage, limited English proficiency), part of most definitions of LD. If the person does not have a cognitive strength or achievement deficit related to a cognitive weakness or meets an exclusion, the person is not identified with LD.
There are five steps in the C-SEP process: (1) Administer the seven core cognitive subtests that represent the seven broad cognitive domains measured by the WJ IV. If there are no cognitive weaknesses, cognitive testing can be discontinued. If there is a weakness, a second measure is administered to confirm the weakness. (2) Administer the four core subtests of the WJ IV Tests of Oral Language. If there are no deficits, testing is discontinued. If there is a deficit, more testing is completed to assess possible oral language difficulties. (3) Administer six core subtests of the WJ IV Tests of Achievement. Additional achievement testing can be performed to address the referral question. (4) Ensure that the academic problems are not primarily due to the exclusionary factors. (5) Conduct an integrated data analysis that includes testing data, other criterion-referenced testing data, progress-monitoring data, and other informal indices or performance indicators (grades, teacher reports, student observation). In the C-SEP method, the key issue for weaknesses is scores that are “below average.” A strength is general evidence of average levels of performance. If the WJ IV is used, the determination of strengths and weaknesses can be made with the scoring software for the cognitive, oral language, and achievement tests.
Although Cheramie et al. (2018) and Schultz and Stephens (2015; 2017) view C-SEP as a variation on DD/C, there are key differences, some of which may affect the reliability of decisions emerging from the two approaches. Less testing is associated with the C-SEP because the DD/C approach requires two subtests per domain. The determination of strengths and weaknesses in the DD/C approach requires proprietary software, and the C-SEP uses the WJ IV scoring software. Additionally, the C-SEP formally tests oral language, which is not a prescribed part of the DD/C approach. Both approaches emphasize the need to include considerations beyond test scores, especially in consideration of exclusionary factors and informal assessments. Cheramie et al. present two case studies to demonstrate that similar decisions would be made if C-SEP were used instead of DD/C, with less testing.
Implications
There is no research base on the C-SEP. Cheramie et al. (2018) present no evidence demonstrating the reliability and/or validity of decisions based on the C-SEP. However, if studied, this approach would likely present the same problems associated with other PSW methods (see Fletcher, Lyon, Fuchs, & Barnes, 2018, and Phipps & Beaujean, 2016, for a thorough review of problems with the reliability and validity of cognitive discrepancy methods, including IQ-achievement and PSW methods). More generally, like other proponents of PSW methods, Cheramie et al. argue that the Individuals with Disabilities Education Act of 2004 (IDEA) requires cognitive assessments for identification because the statutory definition indicates that LD is “a disorder in one or more of the basic psychological processes involved in understanding or in using language, spoken or written, which may manifest itself in an imperfect ability to listen, speak, read, write, spell, or to do mathematical calculations” (U.S. Office of Education, 1968, p. 34). However, the critical focus of this definition is the manifestation of these psychological processing disorders in imperfect academic abilities. This emphasis on the manifestation of academic achievement problems is clearly apparent in the regulatory definitions that the Department of Education produced for operationalizing this statute. Cognitive processing assessments are not required; the guidance to implementation for IDEA 2004 plainly stated: “The Department does not believe that an assessment of psychological or cognitive processing should be required in determining whether a child has an SLD. There is no current evidence that such assessments are necessary or sufficient for identifying SLD. Further, in many cases, these assessments have not been used to make appropriate intervention decisions” (Individuals with Disabilities Education Act Regulations, 2006, p. 46651).
The evidence offered for the reliability and validity of PSW methods is indirect. Proponents cite four types of evidence in support of these methods, representing research on (1) the structure of cognitive functioning, (2) the relations between cognitive functioning and academic achievement, (3) the reliability and validity of the tests themselves, and (4) the need to identify potential aptitude by treatment interactions. However, this research does not directly support the validity of LD identification decisions based on C-SEP or DD/C. When these decisions are examined for PSW methods, there is little evidence that children with low achievement who meet PSW criteria can be meaningfully differentiated from children with low achievement who do not meet PSW criteria. Most significant for Child Find issues is that PSW methods identify very low numbers of children with LD. If the decision is “yes LD,” the false positive rate is very high, although the false negative rate for “no LD” is very low. Additionally, the methods are not interchangeable. Despite the case studies, it is likely that if subjected to an empirical evaluation, C-SEP and DD/C would identify different groups of low achievers with and without LD. PSW status (and cognitive assessments more generally) do not enhance prediction of treatment response, and there is no evidence that knowledge of the PSW profile facilitates treatment decisions. In summary, these methods recommend a lot of expensive testing to identify a small number of people inaccurately and with no enhancement of treatment outcomes.
PSW methods are typically completed as part of the comprehensive evaluation required by IDEA for special education services. Cheramie et al. (2018) rightly note that other criteria need to be used to make identification decisions. We would argue that testing should be minimized to norm-referenced achievement tests, evaluations of instructional response, and assessments related to the exclusionary criteria that often represent other problems or contextual factors requiring intervention. This approach, which we have termed a “hybrid method,” can be shown to be reliable and valid (Fletcher et al., 2018) and cost effective because it minimizes formal assessment to a short battery of norm-referenced achievement tests, assessments of instructional response that should already be available, and contextual factors and other problems that vary with each assessment.
Cognitive assessments are not obviously necessary for LD identification, a fact illustrated by the two case studies presented by Cheramie et al. (2018). In the first case study, a child with basic reading problems is described. In the hybrid method we have described, we can ignore the cognitive and language testing data and examine the achievement testing, as well instructional response and exclusionary criteria. The child has word reading and spelling scores below the 10th percentile and reading comprehension and writing scores below the 20th percentile—a score profile clearly associated with possible dyslexia. The student’s average math skills eliminate the possibility of an intellectual disability. Unfortunately, the case study provides little information on her instructional history, including the type of core reading program she experienced in general education, any interventions that were provided, and her response to those interventions. There is also no information on her emotional response to her reading difficulties, motivation, teacher reports, and other information essential to developing a treatment plan. By virtue of the nature of her reading problem, we know she needs an approach that teaches decoding explicitly through phonics, fluency building practice, spelling work, and assistance integrating these concepts with comprehension. The cognitive data, which show expected associated deficits in phonological processing and working memory, do not add vital information to the treatment plan. What would be different if she had this type of reading problem and did not have a cognitive strength or weakness in the expected direction?
The second case demonstrates divergence between C-SEP and DD/C, largely because the C-SEP approach did not find a cognitive weakness, which was apparent in DD/C. Again, the question is what difference the presence or absence of the cognitive strength or weakness makes in determining intervention. The featured child has a score in computational arithmetic below the 10th percentile with average reading and spelling performance and adequate performance on a measure of math word problems. In this case study, we learn that he has received adequate instruction and did not meet state benchmarks in math, which is surprising because most state tests are based largely on word problems. There is no obvious processing weakness, except potentially in working memory (number series), which was identified by DD/C. In Step 5, it is noted that additional assessment may be needed. For example, there is no assessment of attention-deficit hyperactivity disorder, with teacher ratings of inattention often strongly related to arithmetic performance (Fuchs et al., 2005). Inattention may explain his lower performances on number series and visual-auditory learning because both require sustained attention, but attention-deficit hyperactivity disorder is not identified based on cognitive tests—it is a historical diagnosis, and teacher and parent ratings are helpful. Regardless of the cognitive pattern, the intervention program would focus on explicit instruction in math facts and computations with a major component involving practice and feedback. There seem to be discrepancies between C-SEP and DD/C, but the discrepancy is in cognitive processing, not academic achievement. Again, what does the pattern of strengths and weaknesses add to the identification of an academic deficit that was associated with real-life consequences: failure on the state test? There would be no difference in the intervention plan or in the need for additional assessment, but the C-SEP assessment would presumably not make the child eligible for these interventions through special education.
Conclusions
C-SEP has no research basis as an indicator of LD status or in relation to intervention. Like other PSW methods, there is no reason the think that the problems associated with these methods would not apply to the C-SEP approach. IDEA indicates that any method adopted for identification of LD must be research based. Like other PSW methods, C-SEP has not established this research base. We encourage identification based on assessments that are related to academic treatments and that prioritize intervention over identifying the right child with “true LD,” a task no PSW method has proven it can reliably achieve. Absent evidence for the reliability and validity of these PSW methods, the high costs associated with cognitive assessment would be better used implementing evidence-based interventions.
References
Flanagan, D. P., Alfonso, V. C., Sy, M. C., Mascola, J. T., MacDonough, E. M., & Ortiz, S. T. (2018). Dual discrepancy/consistency operational definition of SLD: Integrating multiple data sources and multiple data gathering methods. In D. Flanagan & V. Alfonso (Eds.), Essentials of specific learning disability identification (2nd ed., pp. 329–430). Hoboken, NJ: John Wiley & Sons.
Fletcher, J. M., Lyon, G. R., Fuchs, L. S., & Barnes, M. A. (2018). Learning disabilities: From identification to intervention (2nd ed.). New York, NY: Guilford Press.
Fletcher, J. M., & Miciak, J. (2018). A response to intervention approach to SLD identification. In D. Flanagan & V. Alfonso (Eds.), Essentials of specific learning disability identification (2nd ed., pp. 221–257). Hoboken, NJ: John Wiley & Sons.
Fuchs, L. S., Compton, D. L., Fuchs, D., Paulsen, K., Bryant, J. D., & Hamlett, C. L. (2005). The prevention, identification, and cognitive determinants of math difficulty. Journal of Educational Psychology, 97, 493–513.
Hale, J., Alfonso, V., Berninger, V., Bracken, B., Christo, C., Clark, E., . . . Yalof, J. (2010). Critical Issues in response-to-intervention, comprehensive evaluation, and specific learning disabilities identification and intervention: An expert white paper consensus. Learning Disability Quarterly, 33, 223–236.
Phipps, L., & Beaujean, A. (2016). Review of the pattern of strengths and weaknesses approach in specific learning disability identification. Research and Practice in the Schools, 4, 18–28.
Schultz, E. K., & Stephens, T. L. (2015). Core-selective evaluation process: An efficient & comprehensive process to identify students with SLD using the WJ IV. Journal of the Texas Educational Evaluators’ Association, 44, 5–12.
U.S. Office of Education. (1968). First annual report of the National Advisory Committee on Handicapped Children. Washington, DC: U.S. Department of Health, Education, and Welfare.