Kopatich, R. D., Magliano, J. P., Millis, K. K., Parker, C. P., & Ray, M. (2018). Understanding how language-specific and domain-general resources support comprehension. Discourse Processes. Advance online publication. doi:10.1080/0163853X.2018.1519358
Summary by Dr. Yusra Ahmed
Overview
In an effort to enhance scientific understanding of reading comprehension, several models for organizing the different processes and components underlying proficiency in reading comprehension have emerged. These models include the Landscape Model, Construction-Integration Model, Structure-Building Framework, Verbal-Efficiency Theory, and Reading Systems Framework (Fletcher, Lyon, Fuchs, & Barnes, 2018). More recently, there is an increasing effort to empirically validate theoretical concepts and higher-order cognitive processes proposed by these models by (a) increasing the precision with which concepts such as inference making are measured and (b) carefully modeling the relations among components and reading comprehension in typically developing students and students with reading difficulties.
Direct and Inferential Mediation Model
The Direct and Inferential Mediation (DIME) model of reading comprehension (Ahmed et al., 2016; Cromley & Azevedo, 2007; Cromley, Snyder-Hogan, & Luciw-Dubas, 2011) is an example of an empirical model that treats specific processes involved in reading comprehension as a set of interrelated components. An empirical model refers to a statistical model based on experimental or correlational data rather than theory alone. The DIME model hypothesizes relations not only between component skills and reading comprehension, but also between these component skills themselves: (1) word reading, (2) vocabulary, (3) background knowledge, (4) strategies, and (5) inference (see Figure 1 for a schematic of the DIME model). All component skills have a direct effect on reading comprehension. In addition, two sets of indirect relations are specified: (a) the effect of vocabulary and background knowledge on inference making is mediated by reading strategies and (b) the effect of vocabulary and background knowledge on reading comprehension is mediated by strategies and inference making. In this way, the model hypothesizes mechanisms by which one component affects another.
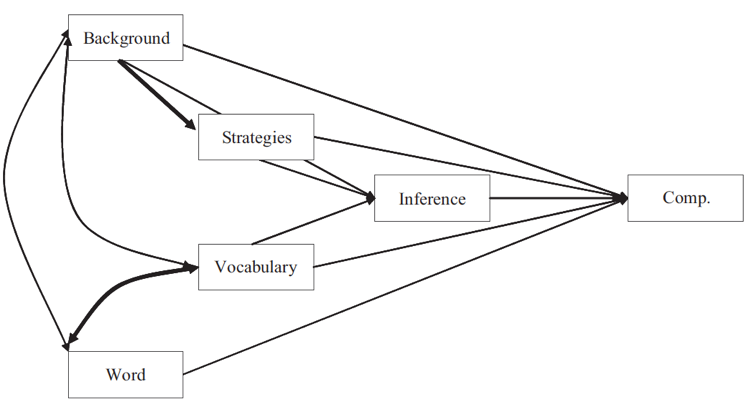
Figure 1. Illustration of the DIME Model using observed variables (Cromley & Azevedo, 2007).
Key findings from prior research on the DIME model using large and diverse samples of students in middle school through college have found that the well-established relations between reader resources (word reading, word knowledge/vocabulary, and world knowledge/background knowledge) are mediated through inferencing (Ahmed et al., 2016; Cromley & Azevedo, 2007; Cromley et al., 2011). That is to say, word reading, word knowledge, and world knowledge influence inference making, which in turn influences reading comprehension. Inferencing refers to the ability to make connections between text ideas or between background knowledge and the text when explicit connections are not provided. Inferences are necessary to establish a coherent understanding of text. Specifically, readers iteratively construct and update mental models, or representations of what is depicted and implied by the text, to establish causal connections. For example, after reading sentences 1 and 2 below, good readers will be able to infer sentence 3.
- Sentence 1: Jane took an aspirin.
- Sentence 2: Her headache went away.
- Sentence 3: Aspirins relieve headaches.
Inferential Mediation Model
Inspired by the DIME model, Kopatich, Magliano, Millis, Parker, and Ray (2018) developed the Inferential Mediation Model (IMM), which focuses specifically on the relations among two types of inferences (bridging and elaborative) and a specific aspect of reading comprehension (the quality of mental models; see Figure 2 below). Elaborative inferences (knowledge-to-text connections) involve incorporating background knowledge within the mental model. Bridging inferences (text-to-text connections) involve connecting text ideas. By comparison, previous research included both text-to-text and knowledge-to-text inferences under the same construct in the DIME model (Ahmed et al., 2016).
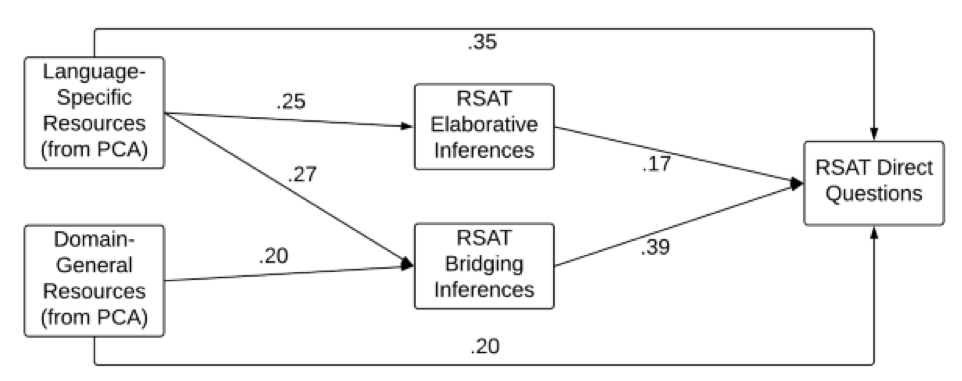
Figure 2. Illustration of the IMM (Kopatich et al., 2018).
Note. Language-specific resources refers to vocabulary, exposure to reading, and reading comprehension. Domain-general resources refers to working memory capacity, meta-cognitive strategies, and need for cognition. Direct questions refers to quality of mental models.
In addition to language processes, Kopatich and colleagues were interested in evaluating the role of domain-general processes (working memory, metacognitive awareness, and need for cognition). They hypothesized that to establish a coherent mental model, readers rely on a variety of domain-general cognitive resources in the same way that they rely on domain-specific linguistic resources. That is, domain-general resources make direct contributions to reading comprehension and indirect contributions through inferences.
In the IMM, language-specific resources were grouped together. This grouping of linguistic knowledge into a single factor aligns with previous research on the DIME model, which found that vocabulary and background knowledge represent a single underlying construct (i.e., they are highly correlated and measure similar skills; Ahmed et al., 2016). Of note, in the IMM model, word reading was not included, reading strategies were grouped with domain-general cognitive processes, and a standardized measure of reading comprehension was included under domain-specific resources.
Method
This study used archival data on 148 college students enrolled in an introductory psychology course, for whom gender and demographic information were not available. However, the sample was likely representative of the larger student population, which was 49% female, 57% white, 16% black, 15% Hispanic, and 5% Asian.
Previous research on the DIME model has used multiple standardized and experimental measures for each component skill to form latent variables. In addition, the studies have included “contextualized” measures of vocabulary and background knowledge (Ahmed et al., 2016); vocabulary, background knowledge, and inferences (Cromley & Azevedo, 2007); and word reading, vocabulary, background knowledge, strategies, and inferences (Cromley et al., 2011) in that measures of component skills were directly related to the content of the comprehension outcome. For example, the topic of the reading comprehension passage used by Cromley and colleagues (2011) was the vertebrate immune system. Accordingly, all other measures also referred to the same passage and topic.
Kopatich and colleagues take “contextualized” testing a step further by evaluating the IMM model during online comprehension. That is, the inference tasks did not rely on multiple-choice questions. Rather, Kopatich and colleagues used think-aloud verbal protocols to provide an assessment of inferences and mental models that were generated during reading six short texts. Think-aloud methodology requires students to vocalize their thought processing periodically while reading the text. Unlike standardized measures of reading comprehension and reaction-time measures of inference making, think-aloud methods provide an assessment of the conscious, mental representations of text.
For inference making and mental model tasks, one sentence was presented at a time on a screen and transitions to new paragraphs were indicated. Previous sentences were not available to students when reporting their thoughts on the text or when writing the answers to direct questions about the text. Thus, their measure of reading comprehension was a departure from standardized measures of reading because it required students to answer how and why open-ended questions designed specifically to be sensitive to the quality of mental models. The verbal protocols were analyzed for bridging inferences (whether people mentioned concepts and clauses from the prior text) and elaborative inferences (whether inferences contained concepts not mentioned in the text), and answers with the correct usage of verb clauses were scored higher. For the direct questions, written responses were scored using a 4-point rubric that ranged from a complete/correct answer to incomplete/incorrect.
Measures of domain-general resources included meta-cognitive strategies (a self-report measure of awareness of reading strategies), working memory span (measured as the number of letters students could recall after deciding whether mathematical operations were true), and need for cognition (a self-report measure of students’ tendency to engage in and enjoy thinking).
Measures of language-specific resources were chosen based on relevance for postsecondary readers. These measures included vocabulary and exposure to text (measured as the knowledge of famous authors). In addition, the authors included the Gates-MacGinitie Test of Reading Comprehension, a standardized test of reading comprehension, as a component of the language-specific resources because they were interested in the effect of comprehension in general on a specific aspect of the reading comprehension process.
Principal components analysis was used to determine whether measures that are theoretically domain-general and language-specific share common variance. The results for these analyses supported the hypothesized two-component solution. These two resource factors were then used as variables in subsequent path analysis models.
Conclusions
Kopatich and colleagues evaluated the role of language-specific and cognitive resources that students bring to bear when reading a text. They replicated the DIME model using a more contextualized measure of reading comprehension in the IMM. Their analyses evaluated the role of bridging vs. elaborative inferences and found that reader resources affect comprehension directly and indirectly via inferences. Thus, their findings support the claim that reader resources alone are not sufficient to support reading comprehension and that engagement with text is necessary. Prominent theories of mental model construction propose that the status of the mental model is updated as each sentence is read to incorporate new content. The mental model stabilizes once all sentences are read. Thus, the current study brings the DIME model more in line with theoretical models of comprehension, which focus on comprehension as a dynamic process rather than a static skill.
References
Ahmed, Y., Francis, D. J., York, M., Fletcher, J. M., Barnes, M., & Kulesz, P. (2016). Validation of the direct and inferential mediation (DIME) model of reading comprehension in grades 7 through 12. Contemporary Educational Psychology, 44, 68–82.
Asendorpf, J. B., Conner, M., De Fruyt, F., De Houwer, J., Denissen, J. J., Fiedler, K., . . . Perugini, M. (2013). Recommendations for increasing replicability in psychology. European Journal of Personality, 27(2), 108–119.
Cromley, J. G., & Azevedo, R. (2007). Testing and refining the direct and inferential mediation model of reading comprehension. Journal of Educational Psychology, 99(2), 311–325.
Cromley, J. G., Snyder-Hogan, L. E., & Luciw-Dubas, U. A. (2010). Reading comprehension of scientific text: A domain-specific test of the direct and inferential mediation model of reading comprehension. Journal of Educational Psychology, 102(3), 687–700.
Fletcher, J. M., Lyon, G. R., Fuchs, L. S., & Barnes, M. A. (2018). Learning disabilities: From identification to intervention. New York, NY: Guilford.
Interested in Going Deeper on This Subject?
Reviewer Dr. Yusra Ahmed also conducted a modeling exercise in which she used the data from the Kopatich et al. (2018) article to fit alternative models. She presents her methods, results, and key findings below.
Modeling Exercise
Replicability and reproducibility are important for building robust theories that are generalizable in different contexts and populations and that can provide decisive guidance for educators (Ahmed et al., 2016; Asendorpf et al., 2013). Because prior research on the DIME model has used the Gates-MacGinitie Reading Test as the outcome measure of comprehension rather than a predictor variable, for this review of the Kopatich et al. (2018) article, a modeling exercise was conducted using summary data (i.e., means, standard deviations, and correlations) from the study to fit alternative latent variable models. Principal components analysis and factor analysis modeling typically yield similar substantive conclusions. Thus, the reproducibility of the IMM model and further modifications using structural equation modeling were conducted to evaluate whether results would change as a function of model specification. The first model replicated the IMM as a baseline model (see Figure 3). The structural model was the same as in Figure 2. Thus, paths from domain-general resources to bridging inferences were excluded, as were correlations or paths between student resources and between inferences. In Figures 2 to 6, the standardized solution is presented and the effects can be interpreted as regression coefficients. This model explained 61% of the variance in reading comprehension. Similar to the original IMM model, language-specific resources predicted both types of inferences and reading comprehension, domain-general resources predicted bridging inferences, and bridging inferences predicted reading comprehension. In the replication, the effect of domain-general resources (dgr) on comprehension (rdq) and elaborative inferences (re) on comprehension were not significant.
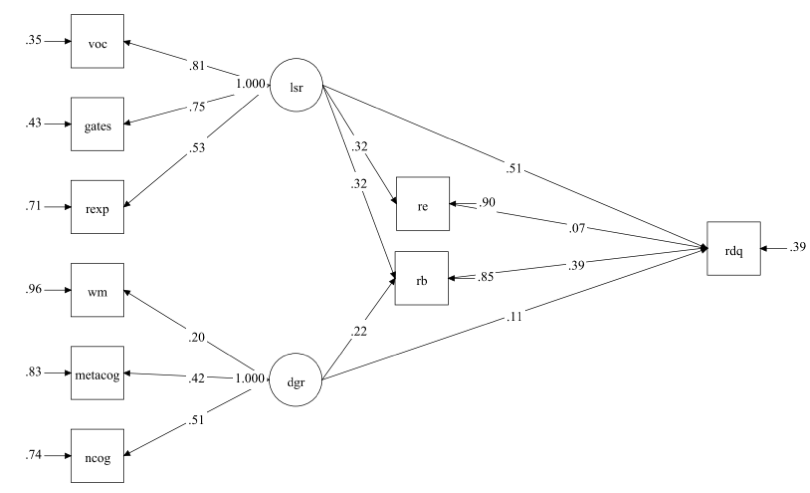
Figure 3. Replication of the IMM model.
Note. Coefficients ≤ .20 were not significant; all other coefficients were significant at p < 0.01. Model fit indices: AIC = 6393.90; Adjusted BIC = 6388.54; Chi-Square = 42.05 (p < 0.01); degrees of freedom = 22; RMSEA = 0.08; CFI = 0.93; TLI = 0.88; SRMR = 0.07. dgr = domain-general resources; gates = Gates-MacGinite Reading Test; lsr = language-specific resources; metacog = metacognitive strategies; ncog = need for cognition; rb = bridging interferences; rdq = RSAT direct question score (reading comprehension); re = elaborative inferences; rexp = reading exposure; voc = vocabulary; wm = working memory capacity.
Next, an alternative measurement model was evaluated in which the Gates-MacGinitie Reading Test was as an indicator of reading comprehension (see Figure 4). Similar to previous studies using latent variables, this model explained virtually all of the variance in comprehension (R2 = 0.999). Only the effect of elaborative inferences (re) on comprehension (rc) was not significant in this model. The model in Figure 4 was statistically significant from the baseline model in Figure 3 and provided a better fit to the data.
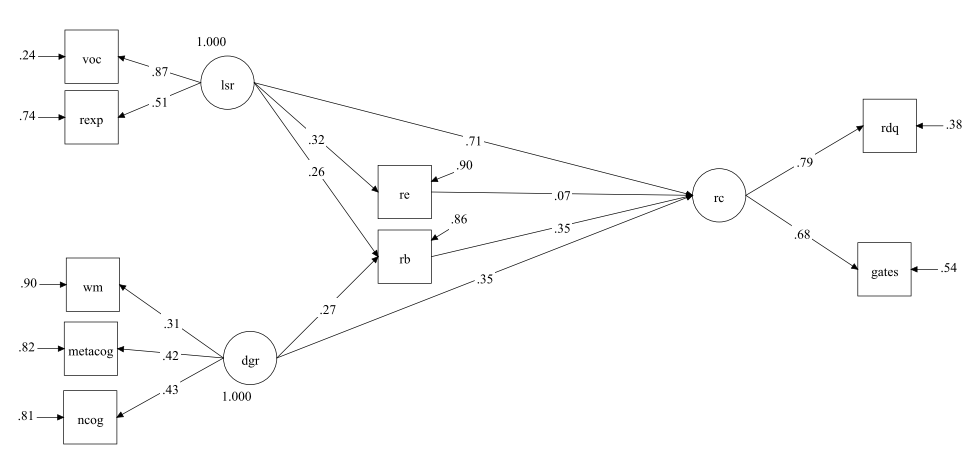
Figure 4. Modification of the IMM using Gates-MacGinitie Reading Test as an indicator of reading comprehension.
Note. Coefficients ≤ .20 were not significant; all other coefficients were significant at p < 0.05. Model fit indices: AIC = 6387.23; Adjusted BIC = 6382.04; Chi-Square = 37.38 (p < 0.05); degrees of freedom = 23; RMSEA = 0.07; CFI = 0.95; TLI = 0.92; SRMR = 0.09.
Then, an alternative measurement and structural model was evaluated in which metacognitive strategies were an independent measure of reading strategies and inferencing was a latent variable measured by bridging and elaborative inferences (see Figure 5). In this model, the paths were similar to those in the IMM model and the original DIME model (Figure 1). Thus, paths were specified from domain-general and language-specific resources to strategies and inferences, which in turn predicted comprehension. Compared to the previous models, this model represented a poorer fit to the data. As can be seen in Figure 5, the small loading for elaborative inferences (0.29) indicates that bridging and elaborative inferences did not adequately represent a single underlying dimension. The only significant path coefficient in this model was language-specific resources (lsr) to reading comprehension (rc); all other coefficients were not significant.
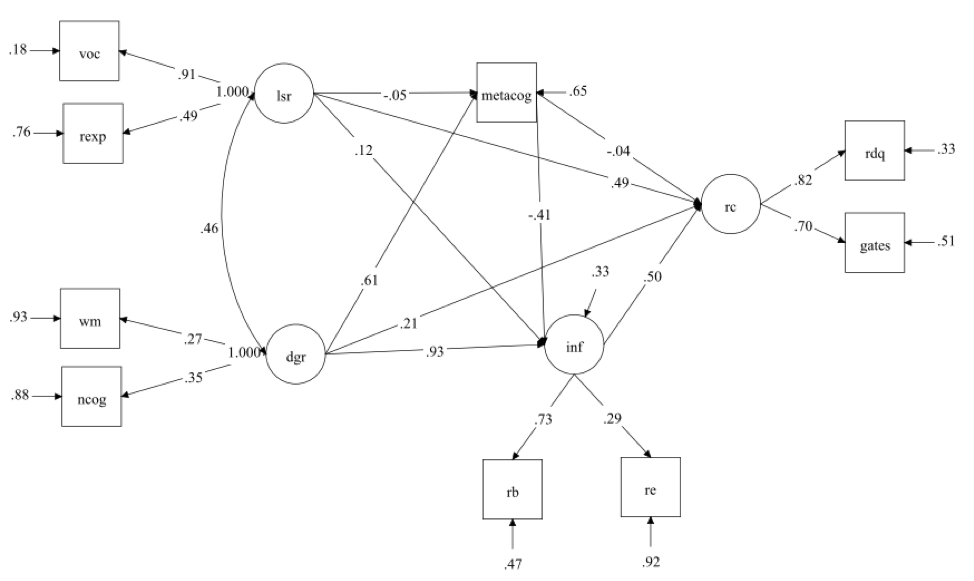
Figure 5. Modification of the IMM using Gates-MacGinitie Reading Test as an indicator of reading comprehension and metacognitive strategies as a measure of reading strategies.
Note. Model fit indices: AIC = 6399.89; Adjusted BIC = 6393.86; Chi-Square = 40.03 (p < 0.01); degrees of freedom = 18; RMSEA = 0.09; CFI = 0.92; TLI = 0.84; SRMR = 0.05.
Finally, to make direct comparisons with prior research on the DIME model, in the last model, domain-general resources were dropped because this construct was not included in the original studies or subsequent replications (see Figure 6). The paths from strategies to comprehension and strategies to inferences (.06) were not significant; all other coefficients were significant at p < 0.05. Similar to the previous models, this model also explained 0.999% of the variance in reading comprehension.
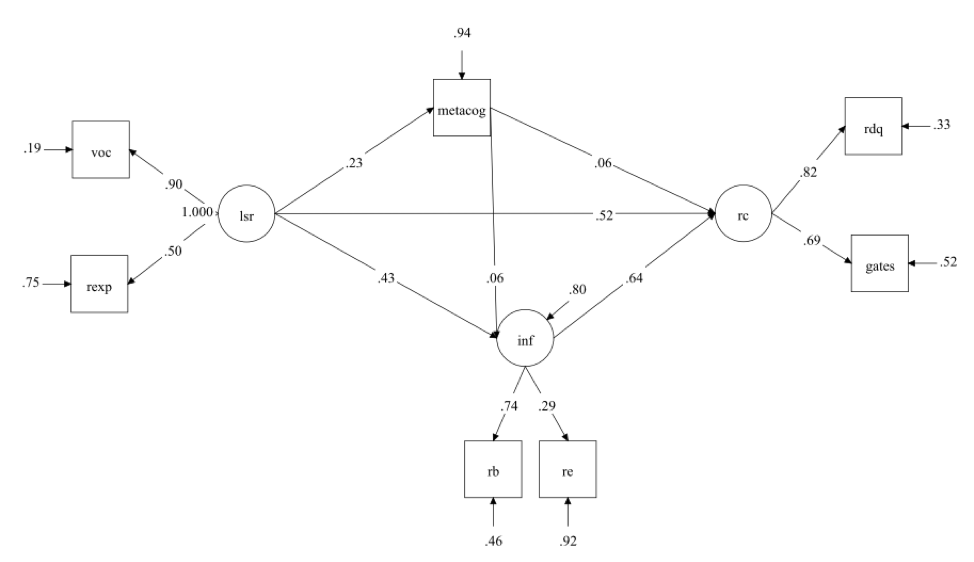
Figure 6. Modification of the IMM using Gates-MacGinitie Reading Test as an indicator of reading comprehension, using metacognitive strategies as a measure of reading strategies, and excluding domain-general resources.
Note. Model fit indices: AIC = 3842.50; Adjusted BIC = 3920.43; Chi-Square = 32.47 (p < 0.001); degrees of freedom = 9; RMSEA = 0.13; CFI = 0.91; TLI = 0.79; SRMR = 0.06.
Key Findings From Modeling Exercise
- Language-specific resources predicted both types of inferences as well as comprehension.
- Domain-general resources predicted bridging inferences but not elaborative inferences, suggesting that bridging inferences are more computationally demanding than elaborative inferences.
- Bridging and elaborative inferences predicted variance in direct question responses, over and above language-specific and domain-general resources.
- Meta-cognitive strategies were categorized as domain-general, cognitive resources rather than language-specific resources, even though this measure of strategies was specific to text.
The current replications supported Kopatich and colleagues’ findings:
- Bridging and elaborative inferences obtained from think-aloud protocols did not represent a single latent trait, but rather two distinct types of inferences.
- Vocabulary and exposure to text represented a single latent variable for language-specific resources in the latent variable framework.
- Working memory, need for cognition, and meta-cognitive strategies represented a single latent variable for domain-general resources.
- Inferences mediated the relations among language-specific variables and reading comprehension. Consistent with previous research on the DIME model, the mediation was through inferential processes rather than through reading strategies.
- Domain-general resources make contributions to reading comprehension, particularly when comprehension is broadly defined.
The current modeling exercise adds to the findings from Kopatich and colleagues in the following ways:
- Bridging inferences are predictive of a general reading comprehension factor, in addition to being predictive of a specific aspect of reading comprehension, quality of mental models.
- Language-specific resources are predictive of reading strategies, but reading strategies measured via self-report were not related to other components of the DIME model.